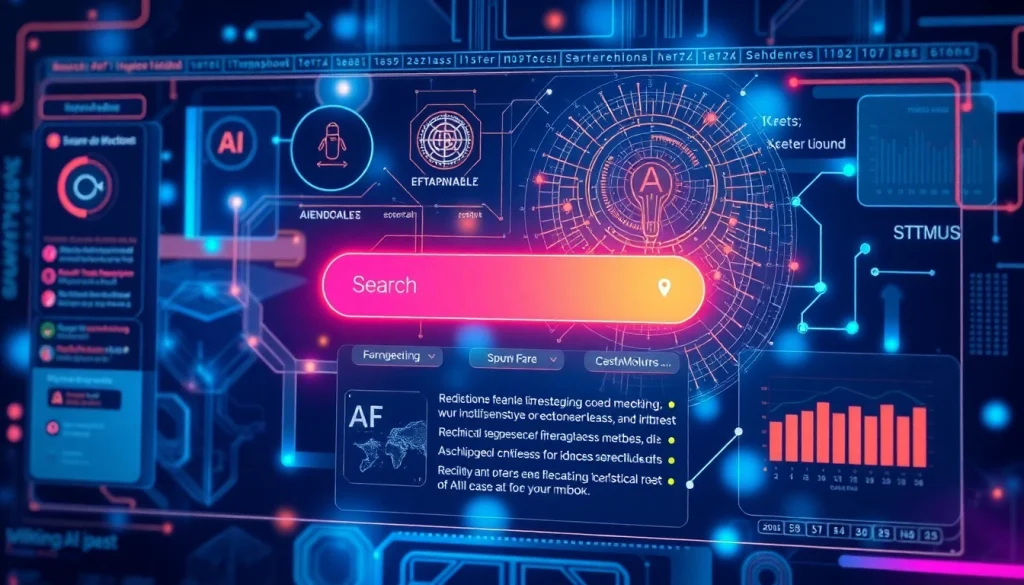
Understanding AI Search Technology
The landscape of digital information retrieval has significantly transformed with the advent of AI search. This innovative approach employs artificial intelligence to enhance the accuracy and relevance of search results, effectively reshaping how individuals and organizations access information.
Definition and Importance of AI Search
AI search refers to technology that utilizes artificial intelligence to improve the efficiency and effectiveness of information retrieval. Traditional search engines often rely on keyword matching, which can produce irrelevant results or overwhelm users with data. In contrast, AI search engines leverage advanced algorithms, natural language processing (NLP), and machine learning to refine the search experience.
The importance of AI search lies not just in its ability to provide more accurate results but also in its capacity to enhance user satisfaction. For businesses, adopting AI search systems can lead to improved customer engagement and retention, as users are more likely to find what they need quickly and effortlessly. This ability to meet user needs is critical in an era where vast amounts of information are generated daily.
How AI Search Works: Algorithms and Mechanics
At the heart of AI search technology are sophisticated algorithms developed through machine learning. When users input a query, the AI system evaluates it against a vast database using various techniques:
- Semantic Search: AI understands the context and intent behind a user’s search query. Rather than merely matching keywords, it analyzes the meaning of words and their relationships to provide more relevant results.
- Natural Language Processing: AI search engines utilize NLP to interpret human language. This involves breaking down the query into its constituent parts to understand the user’s intent better and to handle language variability.
- Ranking Algorithms: AI algorithms prioritize search results based on contextual understanding, user behavior, and previously successful searches. This dynamic ranking ensures that the most relevant and useful information surfaces at the top of the search results.
The Role of Machine Learning in AI Search
Machine learning is crucial to the evolving capabilities of AI search. By analyzing vast amounts of data, these systems learn from user interactions, making them smarter over time. Machine learning enables AI search to:
- Adapt to User Preferences: The more a user interacts with an AI search tool, the better it becomes at anticipating their needs and preferences.
- Improve Result Accuracy: Continuous learning from user feedback helps refine the algorithms, making search results increasingly pertinent to specific user contexts.
- Identify Patterns: Machine learning identifies trends and patterns in user behavior, which can be utilized to enhance the user experience further.
Comparative Analysis of Leading AI Search Engines
A Deep Dive into Google’s AI Search Capabilities
Google has remained at the forefront of search technology for years, and its recent forays into AI search illustrate its commitment to evolving user experience. Google’s introduction of Gemini, a new AI framework, enhances how users interact with the search engine.
Gemini combines capabilities from both text and image searches, allowing users to search by images and receive results that are not just based on keywords but also on the context of visual information. This seamless integration of multimedia search offers a more comprehensive search experience.
Moreover, Google’s AI search algorithms prioritize understanding the user’s context, employing machine learning to refine results based on individual search history and preferences. The result is a more personalized information retrieval process that is effective for both casual users and professionals alike.
Features of Microsoft’s Azure AI Search
Microsoft’s Azure AI Search service presents a powerful option for businesses seeking sophisticated search capabilities. Built on the Azure cloud platform, it integrates powerful search functionalities with machine learning algorithms.
Key features include:
- Retrieval-Augmented Generation (RAG): Azure AI Search incorporates this cutting-edge approach, allowing it to generate answers based on retrieved documents, significantly improving the quality of search results.
- Customizable Search Solutions: Businesses can tailor the search experience to their unique needs, ensuring alignment with organizational goals.
- Integration with Other Azure Services: Seamless operation with Microsoft’s broader ecosystem of Azure services enhances its utility for enterprises seeking a comprehensive solution.
Emerging Technologies in AI Search Platforms
As AI search technology continues to advance, several emerging trends are becoming apparent in the market. These include multimodal search capabilities that allow for the integration of various data types — text, voice, video, and images — into a single search experience.
AI search engines are increasingly adopting techniques such as:
- Voice Search: The rise of smart speakers and voice-activated devices facilitates a shift toward voice searches, necessitating that AI search technologies adapt to understand spoken language nuances.
- Visual Search: Innovations in computer vision enhance capability in searching with images. Users can input images, and AI recognizes objects and retrieves relevant data from across the web.
- Enhanced User Interaction: Chatbot integrations and interactive user interfaces allow users to refine their searches through conversational input, making searches increasingly user-friendly.
Maximizing User Experience with AI Search
Personalization and Relevance in Search Results
One of the hallmarks of AI search technology is its ability to tailor search results to individual users, enhancing the relevance of information provided. Personalization relies on user behavior analysis, including previous searches and interaction patterns.
Innovative features in AI search include:
- User Profiles: Custom profiles created from user interaction history allow AI systems to tailor content, ensuring suggestions are relevant to each individual.
- Contextual Relevance: AI can deduce the context in which a query is made, adjusting results dynamically to cater to situational needs, whether for work, entertainment, or academic research.
Accessibility Features in AI Search
Creating an inclusive search experience involves incorporating accessibility features that cater to diverse user needs. AI search engines have made significant strides in ensuring that everyone can benefit from their tools.
Notable accessibility features include:
- Voice Search Options: Enabling users to speak their queries assists those with visual impairments or other disabilities that make traditional input methods challenging.
- Screen Reader Compatibility: Ensuring that AI search results are compatible with screen reading software enables visually impaired users to access information easily.
- Customized Display Settings: Options for larger fonts, high contrast colors, and simplified interfaces help users with various needs navigate the search experience effortlessly.
Best Practices for Implementation in Your Business
For organizations looking to implement AI search technology, several best practices can maximize benefits and streamline the transition:
- Conduct User Research: Understand your user base, their search habits, and preferences. This insight informs how to tailor AI search functionalities effectively.
- Invest in Training: Educate staff and users on how to leverage AI search tools. Training enhances user adoption and ensures that everyone can benefit from the technology.
- Monitor Performance: Regularly assess how the AI search is performing through analytics and feedback, enabling continuous improvement based on real user data.
Common Challenges and Solutions in AI Search
Data Privacy and Ethical Considerations
As AI search technology becomes more prevalent, data privacy concerns arise. Organizations must be diligent in how they handle user data, especially in light of regulations like GDPR.
Best practices for addressing these concerns include:
- Transparency: Clearly communicate how user data is collected, used, and protected. Users are more likely to engage with AI systems they trust.
- Data Minimization: Only collect data essential for improving search functionality, reducing the risk of data breaches and misuse.
Addressing Bias in AI Search Results
Another critical challenge is the potential for bias in AI search results, stemming from data sets that may not represent diverse perspectives accurately. Algorithms can inadvertently reflect these biases, leading to skewed or unfair search results.
Strategies to mitigate this issue include:
- Regular Audits: Regularly evaluate algorithms and search outcomes for biases, enabling necessary adjustments to ensure fairness.
- Inclusive Data Collection: Develop diverse data sets that better reflect various user demographics and perspectives.
Optimizing AI Search for Multimodal Inputs
As users increasingly utilize various input methods (text, voice, images), optimizing AI search to manage multimodal inputs is crucial for user satisfaction.
Effective strategies include the use of advanced machine learning techniques to ensure the system can understand and process diverse forms of input while maintaining the relevance of search results.
Future Trends in AI Search Technology
Impact of Natural Language Processing on Search
The future of AI search technology is intrinsically linked to advancements in natural language processing. As NLP technology becomes more sophisticated, AI search systems will likely achieve greater accuracy in understanding user intent, leading to more relevant search outcomes.
Future iterations of NLP-enabled AI will facilitate better context recognition, supporting complex queries and allowing for conversational search experiences.
The Rise of Visual and Voice-Activated AI Searches
Expect a substantial increase in visual and voice-activated searches as technology becomes more intuitive. These modes will allow users to engage with AI search in natural manners, rather than through traditional interfaces alone.
The implementation of augmented reality (AR) and virtual reality (VR) technologies may offer immersive search experiences that traditional methods cannot replicate, fostering innovative ways for users to engage with information.
Predictions for AI Search in the Next Five Years
As we look towards the horizon, several predictions emerge regarding the direction of AI search:
- Greater Integration Across Platforms: Expect seamless integration of AI search capabilities into everyday applications, from web searches to business tools and beyond.
- Increased Personalization: The personalization of search experiences will deepen, becoming hyper-relevant and tailored to individual users’ preferences and behaviors.
- Focus on Ethical AI: The rise of ethical considerations will drive organizations to adopt fair, accountable AI search systems, ensuring that search results are equitable and unbiased.